Are we suggesting that applying best practices to supply chain optimization isn’t enough to guarantee data quality? Well. . .yes. (Sorry about that.) Without data metrics in play to quantify performance, your company’s supply chain may not be quite as optimized as you’d like to believe. With more than thirty years of combined experience, our QuadraDot professionals know well the Material Mastering process and what it takes for supply chain optimization.
Big Data is the term used by companies to describe the large volume of data–structured and unstructured–that daily engulfs a business in ever-increasing volumes. In today’s fluid marketplace, a company is only as successful as its ability to make fast, accurate decisions–responding without delay to a fluctuating current of incoming and outgoing data. Without methods and infrastructure in place to measure the exactness of the data stream flowing through your company, unfortunately, things may have already spun out of control.
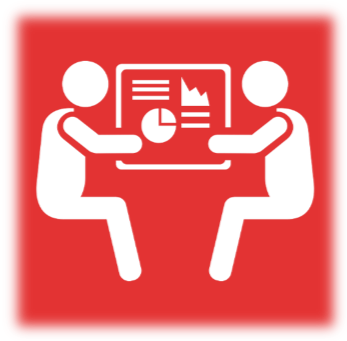
According to information and technology research group Gartner, the financial impact of below-standard data on organizations is an average of $15 million a year. Independent analyst group and London consulting firm Ovum confirms this dismal forecast, saying that poor quality data costs the business community as much as 30% of their total revenue. In light of this, American-based Forrester Research asserts just how essential data analysis is, stating that almost one-third of analysts take more than 40% of their time confirming and assessing predictive analytics data before it can be used for strategic decision-making.
If any of this sounds like the current situation in your company, you may have decided that your Big Data is giving you a BIG headache.
METRICS FOR SUPPLY CHAIN OPTIMIZATION
Supply chain metrics are established by utilizing specific guidelines to quantify and characterize supply chain optimization. Business intelligence software developer Datapine lists ten supply chain metrics that help companies define the perfect ‘sweet spot’ for business performance.
- Cash-to-cash Time Cycle: This metric helps calculate the time required to transform resources into cash flow. Three ratios are utilized—Days of inventory (DOI), Days of payables (DOP) and Days of receivables (DOR). These key performance indicators (KPI), evaluate the time involved between paying cash to a supplier and the moment cash is received from customers.
- Freight Bill Accuracy: Billing accuracy is important for profitability as well as customer satisfaction.
- Perfect Order Rate: This KPI measures the success of a company’s ability to deliver orders incident-free.
- Days Sales Outstanding (DSO): This KPI measures how fast a company is able to collect or generate revenue from customers.
- Inventory Turnover: This logistic KPI helps a business understand the number of times its inventory has been sold within a specific time, which indicates efficient production design, process strategy, fulfillment capabilities and marketing/sales management.
- Gross Margin Return on Investment (GMROI): In supply chain metrics, the GMROI shows a clear indicator of the average investment made in the items in inventory versus the return.
- On-time Shipping: This KPI allows the company to set a benchmark shipping time relative to each product, which promotes streamlining of the entire shipping and delivery process.
- Return Reason: This metric offers insight into the reasons customers give for returning orders, indicating ways to reduce future returns.
- Inventory Velocity (IV): A pivotal KPI, the Inventory Velocity provides a visual snapshot of the percentage of inventory that’s projected for use within the selected time period.
- Inventory Days of Supply: This KPI is the most encompassing of supply chain metrics, giving a fair calculation of the number of days it would take before the company depletes its stock entirely.
TIME METRICS
Cost is the inevitable dynamic when evaluating supply chain optimization, but what about time as an equally important factor when evaluating performance? Here are twelve key areas where the element of time can help or hinder: (Cerasis.com 2019)
- Promise Time
- Cycle Time
- Transit Time
- Delivery Time
- Unloading Time
- Processing Time
- Queue Time
- Quality Assurance Time
- Processing Time
- Turnaround Time
- Receiving Time
- Shipping Time
LEAD TIME METRICS
This brings us to what might be considered the most critical time metric of all: efficient lead times are vital for supply chain optimization.
- How long does it take for a Purchase Order to be rendered once an item is requested? Shortening this process could significantly speed up the procedure.
- Once the Purchase Order has been cut, how long does it take for the vendor to acknowledge and process the order for shipment?
- Is there a way to streamline the shipping process? Could there be a faster way to move particular products?
- A smooth exchange of communication between purchasing and the warehouse impacts the speed of progression—dialog must flow easily in both directions.
- In an emergency situation, are there ways to improve lead time(s) to expedite the process?
Famous Failures in Supply Chain Optimization
MATERIAL MASTERS
Meanwhile, Supply Chain Management is looking for better leverage and spend visibility. Material Masters that are unreliable can result in data that cannot be used in reporting to accurately drive any of the business initiatives forward.
The same or very similar abbreviations often jam Materials Masters and may contribute to unnecessary purchasing spend. Even if companies are aware of potential confusion, such deviations are not easily or efficiently correctable. Running a search query or employing a search formula in spreadsheets can be overwhelming if it is not known precisely where the problem lies.
Big Data is no mystery to our QuadraDot team of professionals. We’ll work with company stakeholders to implement the best solutions to return your data to lean, pristine condition and maintain it going forward using our scalable Granite Material Masters™ software. Our experts will help your company come to understand what we already know: Big Data does not have to equal a big headache.